Driving Change: PeopleSense.AI's Vision for Vulnerable Roadway User Safety
PeopleSense.AI aims to create a holistic integration of technology, human factors, and adherence to regulations, creating a robust and adaptive system capable of proactively addressing critical transportation safety issues of 2024 and beyond. Collaboration with internal and external stakeholders is critical for the success of the approach.
PeopleSense.AI system aims to identify high-risk areas for vulnerable roadway users, recommend safety measures, and facilitate infrastructure improvements by analyzing a comprehensive dataset derived from various transportation-related sources and leverage past relevant experiences of the proposing team.
Project Goal
Harness GenAI to anticipate high-risk areas and generate empirically validated safety measures for vulnerable roadway user safety. The project focuses on capturing spatial-temporal dynamics, uncovering regional trends, and pinpointing critical hotspots to proactively address safety issues. This project goal is in alignment with the vision and mission of the PeopleSense.AI solution from Glocol Networks (Glocol Inc), conceived to address critical needs for innovative approaches in enhancing VRU safety.
The background of lives lost in California is as below:
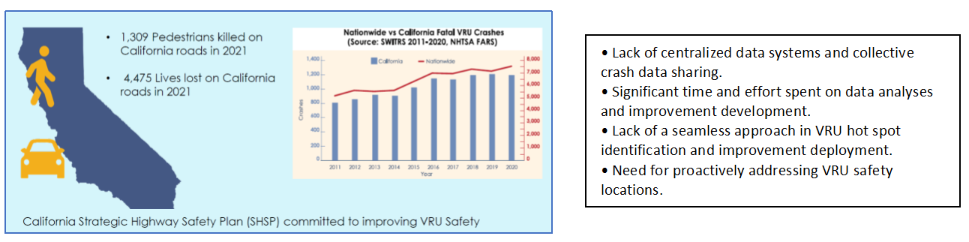
Additionally, Increasing Road Safety has been identified as one of the Critical issues in Transportation for 2024 and beyond, by the Transportation Research Board Executive Committee, TRB, National Academies of Sciences, Engineering and Medicine, so our solution will be a showcase to demonstrate to the Transportation Community.
Core Approach -
1. Data Collection and Deep Learning Techniques
Glocol Networks streamlines the collection of incident and crash data from diverse sources, including CHP SWITRS, CAD, Traffic Volume Data, TSN, Federal Highway Administration Collision Database System, legacy databases, standard accident reports, customer service requests, and other repositories.
This extensive dataset is analyzed using cutting-edge deep learning techniques from PeopleSense.AI to extract valuable insights. By analyzing vast datasets from above sources, the PeopleSenseTM algorithms will identify patterns and predict high-risk locations for VRUs.
Glocol Networks will leverage the learning and experiences gained while deploying deep learning techniques for predictive analysis of high-volume spatial data to identify patterns and predict high-risk areas and data visualization, deployed over a Geographic Information System using ArcGIS, for the various projects and use cases of injecting AI into large traffic and roadway datasets.
2. Proactive Safety Measures
By comparing characteristics of crash sites, such as roadway geometry, traffic and Vulnerable Road User (VRU) behaviors, traffic counts, crash data, speed profiles, and lighting conditions, with similar locations, PeopleSense.AI proactively discerns conflict patterns. This enables transportation operators and engineers to anticipate safety issues in advance and address them more quickly.
3. Safety Recommendation System
The Safety Recommendation System generates recommendations for safety measures and infrastructure improvements for integration into Caltrans’ systems for implementation. A Monitoring & Reporting Module tracks the outcomes of implemented measures and reports on the system’s performance. Stakeholder feedback and system performance data are used to refine and improve PeopleSense.AI predictive capabilities and recommendations.
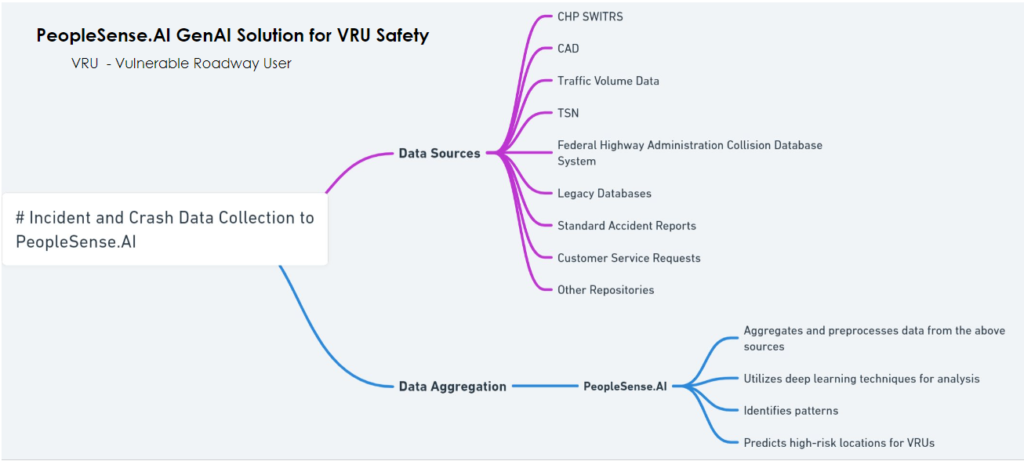
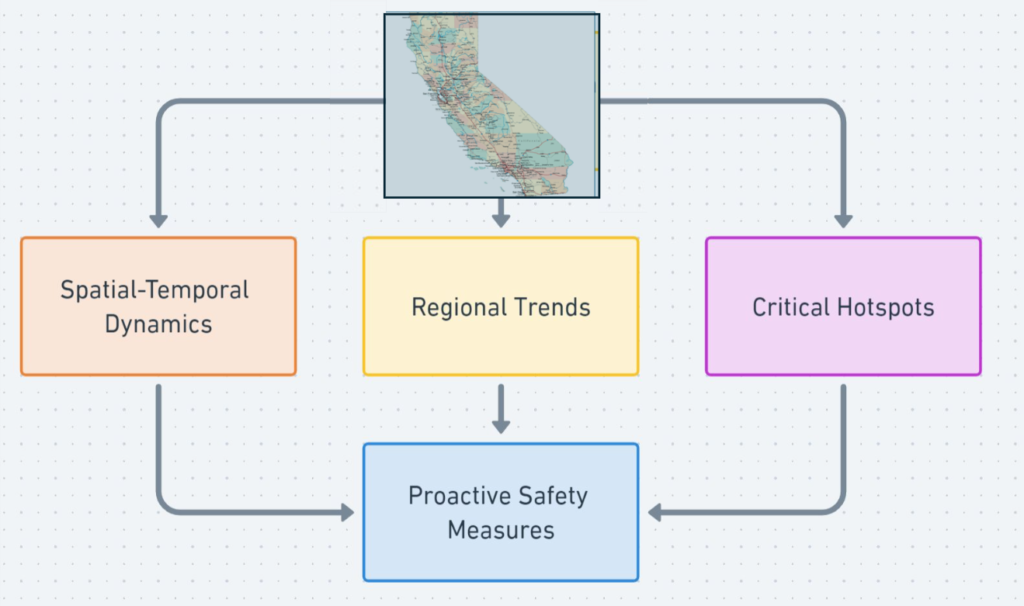
Technology Components and Processes
The proposed solution components and process aligns with the desired requirements:
1. Pre-trained GenAI Solution
The solution will incorporate a GenAI model to analyze and interpret transportation-related data. Examples of models that can be adapted or are directly applicable to transportation-related data analysis:
- Google’s BigQuery ML
- OpenAI’s GPT (Generative Pre-trained Transformer) Series
- DeepMind’s Stream
- H2O.ai
- Facebook Prophet.
- Uber’s Ludwig
- TensorFlow and PyTorch
Some specific pre-trained models are focused on solving time-series forecasting problems, crucial in predicting traffic flow, public transportation demand, or even the availability of shared mobility services, while some models would require collaborative domain expertise to select the right features and train these models on relevant datasets. Additionally, while some models are ready to use “out of the box,” others would require significant fine-tuning and customization to achieve the desired performance on the required transportation-specific datasets and use cases.
2. Integration with Managed Cloud Environments
PeopleSense.AI will ensure seamless integration with required managed cloud environments for efficient and secure operations.
3. Hosting within Managed Cloud Environments
All non-GenAI solution systems, including databases and additional components, will be hosted within a managed cloud environments to ensure centralized management and security.
4. Adherence to Cloud Security Guide
The solution will strictly adhere to the required Cloud Security Guide, implementing robust security measures.
5. Data Pre-processing and Security
The solution will conduct required data pre-processing on state-provided data for tuning the GenAI model, scanning for and blocking sensitive/classified data to ensure compliance and security.
Spatial data formats and other requirements and techniques will be additionally recommended to take full advantage of the Gen AI propositions.
6. Monitoring and Blocking for Sensitive Data
Mechanisms will be put in place to monitor, report, and, if necessary, block GenAI solution prompt inputs for sensitive/classified data and potential harmful prompt methods.
7. Monitoring and Reporting on GenAI Solution Responses
The solution will actively monitor and report on GenAI solution responses for factual accuracy, coherence, and appropriateness, allowing for periodic manual reviews to ensure data integrity.
8. Storage of Input/Output Data
All input and output data generated by PeopleSense.AI will be stored within managed cloud environments, complying with security and compliance requirements
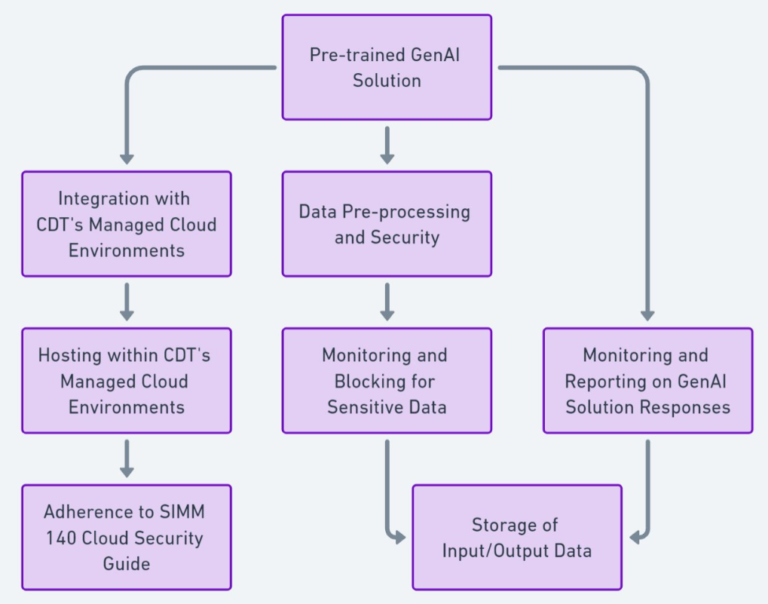
Vision -
To provide GenAI integration for a comprehensive solution that will align PeopleSenseTM algorithms with goals of improving transportation safety, addressing high-risk areas, and facilitating infrastructure improvements.
This outlines a robust framework for enhancing safety on US roadways.
1. THE SOLUTION
Services and Technologies Framework for PeopleSense.AI Solution:
GenAI Model:
A pre-trained, fully-functional Generative Artificial Intelligence (GenAI) model is a core component. This model is designed to analyze and interpret transportation-related data. It contributes to the identification of high-risk areas and the generation of safety measures.
Cloud Services:
Cloud services from the managed cloud environments will be utilized for hosting the PeopleSense.AI system. This ensures efficient and secure operations.
Data Sources:
Databases such as CHP SWITRS, CAD, Traffic Volume Data, TSN, Federal Highway Administration Collision Database System, legacy databases, standard accident reports, customer service requests, and various other transportation-related repositories provide the necessary data for analysis.
Security Tools and Technologies:
Security Tools and technologies will be employed to implement security measures and ensure compliance. This includes adherence to required Cloud Security Guide, which outlines robust security measures.
Monitoring and Reporting Tools:
Tools will be put in place for monitoring and reporting on GenAI solution prompt inputs, responses, and system performance. This ensures factual accuracy, coherence, and appropriateness in the AI-generated responses.
Immediate and Ultimate Solution:
The immediate solution involves the development and integration of the PeopleSense.AI system using a pre-trained GenAI model. The ultimate solution is a proactive and data-driven system capable of identifying high-risk areas, recommending safety measures, and prioritizing infrastructure improvements for transportation safety.
PeopleSense.AI integrates GenAI technologies with deep learning algorithms to analyze real-time and historical data, identifying risk factors and predicting accident-prone areas. The solution comprises three core components:
1. Data Aggregation Engine: Harnesses information from multiple sources, ensuring comprehensive coverage of VRU-related risk factors.
2. Predictive Analysis Module: Utilizes advanced AI algorithms to identify and predict high-risk locations and potential accident scenarios.
3. Safety Recommendation System: Generates actionable insights and data-driven recommendations for infrastructure improvements and safety measures.
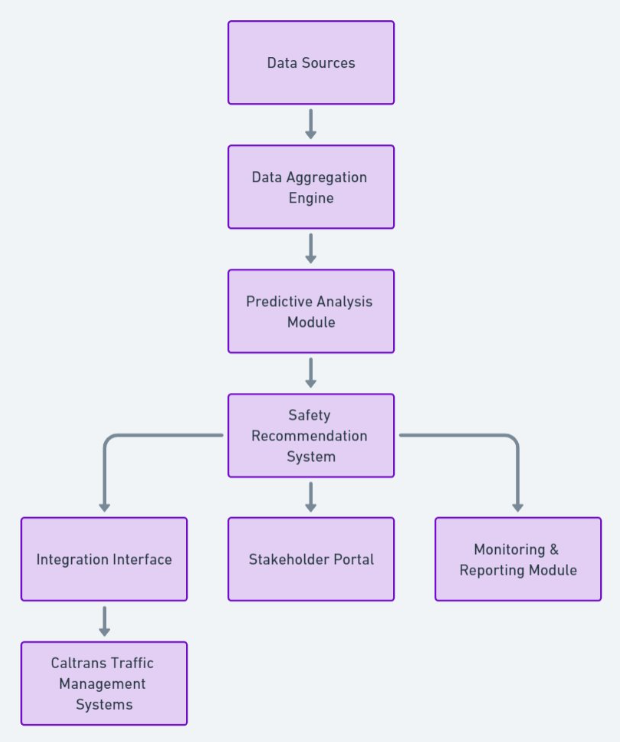
Other Interface components include:
1. Integration Interface: Interfaces for integration with Caltrans’ existing traffic management and planning systems.
2. Stakeholder Portal: A user interface for stakeholders (Caltrans, local authorities, and community organizations) to access insights, reports, and recommendations.
3. Monitoring & Reporting Module: Will track the performance of implemented measures and the overall system, providing reports on effectiveness and areas for improvement.
These components will be developed and deployed as a collaborative effort along with our research partners, ensuring the solution is scalable, adaptable, and equipped with continuous learning capabilities to incorporate new data and evolving urban landscapes.
2. THE APPROACH
Approach for Leveraging the PeopleSense.AI Solution and Addressing the urgent needs of today:
The PeopleSense.AI solution is proposed to be implemented in a phased approach as below:
1. Pilot Phase: Deploy PeopleSense.AI in selected high-risk areas to validate its effectiveness in real-world conditions.
2. Integration Phase: Collaborate with DOT and local authorities to integrate PeopleSense.AI with existing traffic management and planning systems.
3. Scaling Phase: Expand the deployment of PeopleSense.AI across California, prioritizing areas with historically high VRU incidents and underserved communities.
Critical Success Factors and Addressing Them:
Critical success factors include robust seamless integration with existing infrastructure and continuous adaptation to technological advancements as well as ensuring data privacy measures. Performance indicators will include a reduction in VRU-related incidents, improved safety measure implementation rates, and enhanced predictive accuracy.
Integration: The success of PeopleSense.AI relies on seamless integration with diverse data sources, including incident and crash data, gathered from decentralized databases and integration with managed cloud environments. This will be addressed through meticulous planning, testing, and adherence to specified integration standards. Additionally, availability of locational, GIS spatial data and appropriate platforms will be critical for integrating these various data sets.
Human Factors: User training and knowledge transfer are integral. The approach involves comprehensive training sessions for relevant personnel on using and maintaining the PeopleSense.AI system, ensuring optimal human-machine interaction.
Technology: The use of cutting-edge deep learning techniques and a pre-trained GenAI model is a critical success factor. Continuous updates and fine-tuning of the GenAI model ensure optimal performance.
Architecture: The system architecture is designed to accommodate the complexities of spatial-temporal dynamics and diverse data sources. This involves careful planning during the system architecture and development phase.
Interfaces: Interaction with existing databases, cloud environments, and monitoring tools necessitates well-defined interfaces, and this is ensured through adherence to industry standards.
Power and Communications: The solution, being hosted in managed cloud environments, leverages robust power and communication infrastructures for efficient and secure operations.
Existing Regulations: Adherence to the Cloud Security Guide ensures compliance with existing regulations, especially concerning cloud security.
Solution Implementation Location:
The PeopleSense.AI solution will be implemented within managed cloud environments, ensuring centralized management and security. This centralized approach will allow for efficient monitoring and control.
Performance Metrics and Indicators/Success Measures:
Performance indicators include:
- Accuracy of risk identification and safety recommendations.
- System responsiveness to incident and crash data updates.
- Factual accuracy, coherence, and appropriateness in GenAI solution responses.
- Adherence to the Cloud Security Guide.
- Evaluation framework, Including baseline data, target outcomes and a timeline for achieving these performance metrics will be created.
User Training and Adoption: The team will detail a plan for user training, knowledge transfer and system adoption for various operators and other end-users, which will be crucial for the success of the solution implementation.
Potential Partners, Relevant Experiences and Stakeholders:
Internal Stakeholders:
- Relevant decision-makers and transportation operators.
- IT personnel involved in system integration and maintenance.
External Stakeholders:
Stakeholders for cloud environment integration.
- GenAI providers for continuous model updates and support.
- Law enforcement agencies providing incident and crash data.
- Local government authorities for regional collaboration.
- Road infrastructure maintenance and planning authorities.
For more info – reach out by email to info@PeopleSense.AI